Humanoid robots are here to stay, but there are still considerable challenges to be solved before a full robot revolution.
I don’t know about you, but there’s something quite unnerving about Figure 01, the eponymous autonomous humanoid creation of robotics startup Figure. Maybe it’s the blank iPhone-like face, maybe it’s the form-fitted wetsuit. Maybe it’s the sense of latent menace.
This feeling has a name. In the 1970’s, Masahiro Mori, then a professor at the Tokyo Institute of Technology, observed that as robots appear more humanlike, some observers’ emotional response to the robot becomes increasingly positive and empathetic, until it reaches a point beyond which the response quickly becomes strong revulsion. He called this bukimi no tani, literally translated as ‘uncanny valley’.
Well, the uncanny valley is coming to a manufacturing facility near you. Earlier this month BMW, announced a ‘first-of-its-kind’ deal with Figure to deploy their autonomous humanoids at one of the car-maker’s factories in Spartanburg, South Carolina.
Robots in factories aren’t anything new, but they’ve mostly been single-purposed machines only capable of performing specific preset tasks. Humanoid robots promise a new level of adaptability and versatility.
Unlike their single-function counterparts, humanoid robots are designed to mimic human capabilities, featuring advanced hands, arms, and legs that enable them to handle a variety of tasks with agility and precision. They are the same shape as us, they can access the same spaces and use the same tools.
Digit from Agility Robotics has nimble limbs and a torso packed with sensors that enable it navigate complex environments and be used as a “mobile manipulator” carrying bins and empty totes around in warehouses where there’s not enough space for conveyor belts.
EVE from 1X comes with strong grippers for hands, cameras that support panoramic vision and two wheels for mobility. EVE’s are reportedly already working as security guards at a couple of industrial sites in Europe and the US.
Phoenix from Sanctuary has proprietary haptic technology and human-like hands and arms which gives it dexterity to complete tasks that range from stocking shelves and unloading trucks to running registers.
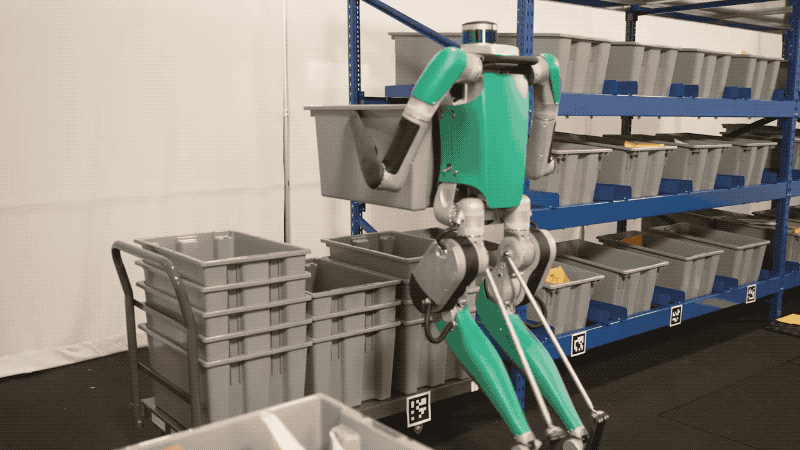
These early models might move like marionettes and have the agility of geriatrics, but it’s getting easier to imagine a future where robots work side-by-side with their human counterparts, taking on dangerous, tiresome, repetitive tasks and freeing us from the burden of physical work.
That future is still a long way away, despite the BMW-buzz. To get to the point where robots are equal to or greater than their human counterparts, engineers need to solve some of the following, not inconsiderable challenges:
Actuators – these are machine components that achieve physical movements by converting energy into mechanical force. They are effectively the muscles of the machine. Future actuators need to have exceptional dynamic range – able to switch in a moment from powerful, rapid movements, such as lifting weights, to delicate, precise actions, like threading a needle.
Energy – powerful actuators will necessitate robust energy systems. These batteries will need to store and deliver sustained power over extended periods while also accommodating rapid and intense bursts of energy when needed. A high-performing robot should operate for extended durations before requiring recharging or will have batteries that allow for rapid charging cycles to ensure continuous functionality.
Sensors – to emulate human capabilities, robots will need a comprehensive sensor suite encompassing all human senses. This includes vision, hearing, smell, balance, proprioception (sense of how ones body, limbs and muscles are orientated), touch for grasping and collision detection, and even a sense of pain to prevent damage.
Compute – the “brain” necessitates powerful processors, capable of complex tasks, such as image recognition, natural language processing, and spatial awareness. Moreover, the computing architecture must strike a balance between processing speed and energy efficiency to ensure optimal performance without compromising the overall energy budget of the robot.
Control – to navigate the complexities of the world, a robot has to be capable of real-time decision-making, learning from experiences, and adapting to dynamic surroundings. It needs to take inputs from its sensors, make a model of its environment and understand how to orientate itself. It needs to determine its objective and make a decision about how to achieve that objective. It needs to plan its movements inline with its surroundings. It needs to instruct motor controllers to move its limbs to locomote and engage with objects in its space. This measure-plan-move sequence must also continuously adapt as the robot moves through its environment and as people and objects move around it.
To solve these challenges will require considerable breakthroughs in AI, digital electronics, material science and mechanical engineering. Just the energy issue may necessitate an entirely new source?!
One out-there idea is to use biobatteries (energy storage devices powered by organic compounds). The robot would have enzymatic components allowing it to “eat / drink” organic material which would then be converted into energy via enzymes; very similar to how we break down food. Another is to use a radioisotope thermoelectric generator (RTG), a type of nuclear battery that converts the heat released by the decay of a radioactive material into energy.
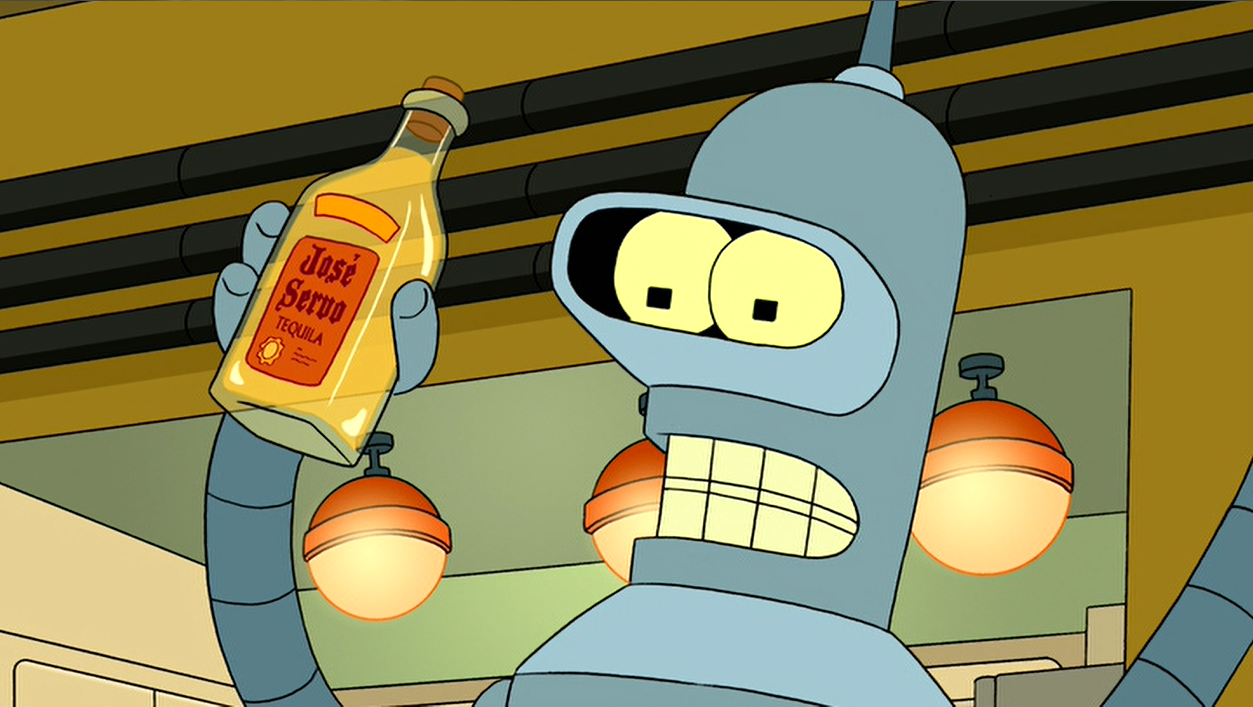
Some advances are happening faster than others. Last year, Toyota Research Institute (TRI) and Columbia University announced the development of Diffusion Policy a new, powerful generative-AI approach that enables easy and rapid behaviour learning from demonstration training.
It’s a big step. Previous state-of-the-art techniques to teach robots new behaviours were slow, inconsistent, inefficient, and often limited to narrowly defined tasks performed in highly constrained environments. Roboticists needed to spend many hours writing sophisticated code and/or using numerous trial and error cycles to program behaviours.
With Diffusion Policy, robots can now watch, learn and replicate what they see. They can be taught more human-like routines and motions without the typical complexity and expense. As an example, Figure (the aforementioned robotics startup) recently demoed Figure 01 making coffee by putting a capsule in a coffee maker.
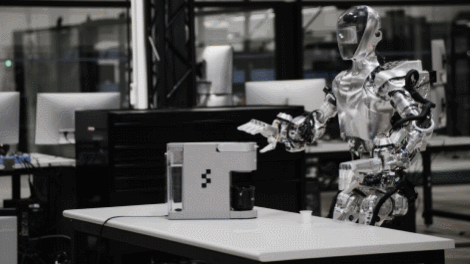
Whilst this might not look particularly impressive – it’s worth noting that the robots end-to-end AI system was trained by watching humans make coffee, in just 10 hours. Not only could it insert the capsule and get the coffee machine started, it also learned to self correct mistakes. To make matters more impressive, this autonomous action is now transferrable to any other Figure robot running on the same system via swarm learning.
These skills are not limited to just “‘pick and place” or simply pushing around objects. Thanks to the groundwork laid by Diffusion Policy robots can now interact with the world in varied and rich ways. There’s still a huge amount of work still to be done, but its a significant step to ultimately building “Large Behaviour Models (LBMs)” for robots – analogous to the Large Language Models (LLMs) that have revolutionised conversational AI.
Till next month.